Episode Highlights:
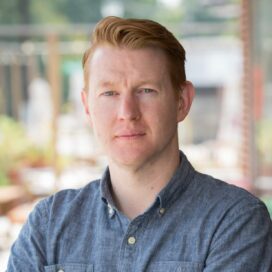
Rich Briddock: “MMM allows you to achieve a similar thing in terms of optimizing your media mix to get a better output, but without having to jump through those hoops of how do I unify Facebook data with Google Ads data with organic data.“
Episode Overview
Lauren Leone and Rich Briddock explore media mix modeling (MMM) and introduce Cardinal’s proprietary solution, RevRx™. They discuss why MMM has re-emerged as a vital tool for guiding marketing investment decisions, especially in healthcare.
Rich explains that MMM is a statistical method to correlate marketing investments with revenue, giving insights into how various channels contribute to overall business growth. Unlike multi-touch attribution (MTA), which can be difficult to implement and unify across platforms, MMM provides a more accessible and comprehensive understanding of the impact of marketing efforts. This is especially crucial in healthcare, where privacy regulations like HIPAA make data tracking more complex.
RevRx™ is positioned as an investment mix model, broader than traditional MMM, incorporating not just media but other factors like SEO and field initiatives. The model helps businesses assess the marginal revenue impact of investing in different channels and tactics, making it easier for marketing leaders to justify investments in channels like Meta or Display, which are often undervalued due to attribution challenges.
As technology advances, MMM solutions like RevRx™ are becoming faster, more affordable, and more adaptable to real-time market changes. Cardinal’s approach emphasizes the importance of aligning marketing investments with business constraints, such as provider capacity, to ensure efficient and scalable growth.
This episode underscores how investment mix modeling can empower healthcare marketers to make data-driven decisions, optimize media spend, and achieve more impactful business outcomes.
Announcer: Welcome to the Ignite Podcast, the only healthcare marketing podcast that digs into the digital strategies and tactics that help you accelerate growth. Each week, Cardinal’s experts explore innovative ways to build your digital presence and attract more patients. Buckle up for another episode of Ignite.
Lauren Leone: Hey, everyone. Welcome back to Ignite: Healthcare Marketing Podcast. My name is Lauren Leone, your Chief Growth Officer. I’m here with my 10-plus years colleague, Rich Briddock, our Chief Strategy Officer. Today, we’re here to talk about media mix modeling. This is something we’ve been getting asked about a lot in recent discovery calls from recent clients. It seems to have made a re-emergence, so we’re going to talk a little bit about why. Most importantly, on today’s episode, we want to introduce you all to Cardinal’s labor of love, our own proprietary investment mix model, which we have called RevRx™. All right, Rich. For those listening in that may not be familiar with MMM, media mix modeling, or what we’re calling investment mix modeling, let’s just give a quick rundown of what it even is.
Rich Briddock: Essentially, it is a statistical way to correlate investment decisions in marketing with revenue that is driven by the business. If I make the following investment decisions in my media mix, my channel mix, you probably heard it called, what is the likely outcome that I’m going to get in terms of the revenue that will be driven for the business. What media mix modeling or investment mix modeling is looking at is not just the impact on revenue that is derived from that investment, but the impact on revenue in totality. It helps you to understand what percentage of your revenue is even driven by your efforts as a marketing team. It is obviously not 100% of your revenue, right? How much of your revenue, assisted revenue that’s driven by your marketing efforts? Then from that baseline number, how can you optimize your media mix to improve the outcome and drive more revenue?
Lauren: I think you made a great point in talking about correlation. What MMM is not is multi-touch attribution. It is not this dollar went in and produced this dollar out. It is the correlation between a set of activities and they don’t have to be media only and the impact that has on your overall revenue.
Rich: Yes. That’s a massive advantage, right? Because I’m sure a lot of people listening to this podcast, 7 out of our 14 listeners will have tried to dabble with multi-touch attribution in the past, or will have approached it as a topic, as something that they want to get into, only to be faced with a myriad of challenges, right? When you think about having a more diverse media mix, so if you’re running meta ads, if you’re running TikTok, if you’re running programmatic, a lot of conversion activity for those platforms happens as a view through as an impression-based conversion. It’s very difficult to track that in a universal model. Also those platforms have different attribution models, so it’s very difficult to unify into a single attribution model.
MTA is almost a non-starter for most companies. Unless you’re willing to invest serious, serious manpower, serious, serious amounts of money, you’re just never going to get to that perfect solution that multi-touch attribution promises. MMM allows you to achieve a similar thing in terms of optimizing your media mix to get a better output, but without having to jump through those hoops of how do I unify Facebook data with Google Ads data with organic data. Right.
Lauren: What we’re trying to do is help. For those of you listening, if you’re a marketing leader in your organization and you’ve really struggled to get out of, let’s say, the search universe because nothing else converts at the same rate or you can’t attribute at the same rate, your cost per acquisitions aren’t the same on Meta or Display, and your tendency is to continue to turn those channels off because the person giving you the dollars is saying this isn’t efficient, this is another mechanism through which we can help C-levels understand the impact of some of these upper funnel, maybe more traditionally branding initiatives, and that they are driving actual revenue for the business.
Rich: Right. Because you can actually see their influence on the mix and on the revenue that comes out of the bottom. I think what media mix modeling or investment mix modeling brings with it are some concepts, because what it’s really looking at is what is the incrementality that I’m going to drive? What is the incremental revenue I’m going to get when I make these shifts? Not the total revenue so much, but the incremental revenue. What that forces you to look at is the margins and things like the marginal cost per acquisition of a channel that you’re currently running. If you have been 100% search focused, you may have a blended cost per acquisition of $50, and you may say, “I love that. I love that my blended acquisition cost is $50. That’s within my target,” but your least efficient cost per acquisition may be $1,000.
Lauren: The next dollar you invest on that 50 might be–
Rich: The next dollar you invest may be $1,000, right? That might be way above your target, and you’re actually losing money on any additional dollar that you’re putting into search. Whereas if you diversified and went into Social or Display, you may drive $150 cost per acquisition, still worse than the blended acquisition of search, but much better than putting that next dollar into search.That’s the key piece.
Lauren: Why do you think media mix modeling has resurfaced as a topic? What’s driving that?
Rich: I think there’s three reasons. One is what we just touched on, which is MTA feels out of sight for a lot of people. Especially in healthcare. Especially in healthcare, yes. That was going to be the add-on piece I was going to allude to with that is with HIPAA compliancy, tracking has become more difficult. There’s more hoops to jump through. There’s more tech you have to get. Meta is always going to be a wolf garden. You’re never really going to be able to understand what’s going on there from an impression point of view. Similar with programmatic vendors too. There’s very few that you can actually place those view through impressions and those view through conversions into a patient journey and measure it in a holistic attribution model.
Now that you’ve got people shying away from tracking more and trying to track in a more sophisticated way and that’s becoming more expensive in healthcare. Plus at the same time. media mix modeling solutions are getting cheaper, and they’re getting faster, and they’re getting more accessible. You’ve got measurement getting more difficult, media mix modeling getting more accessible, and that’s why I think there’s become more of a shift towards using MMM as the primary investment tool versus multi-touch attribution to try and understand where the next dollar should be invested.
Lauren: When we talk about MMM getting more accessible, we’re talking, historically, you may be required to spend $100,000 a month in the past to be able to access this AI or these algorithms, and now we’re talking maybe 2% of your total marketing dollars, maybe a few thousand dollars a month. It is a big shift.
Rich: Huge shift, and also it used to be that you would engage with a specialist agency or a specialist provider that would do media mix modeling, and they would give you a report maybe once every 90 days or once every six months. The issue with that is everything in the marketing world, and especially in the digital marketing world, shifts so quickly. What happens if you get your media mix modeling output in January, and then Google launches two new products in February, but your data from your January–
Lauren: [unintelligible 00:07:05] output.
Rich: You can’t then make determination about these new channels or pivots in the existing channels that are happening at such a quick pace. That information is out of date, whereas now you can run MMM scenarios with your model in almost real time. “Hey, Google released a new thing, a new product, this is what I anticipate that new product is going to yield.” Maybe you run a 30-day test to see what you think it’s actually going to yield for you. Then you can put that in to the model as a factor. It can then take that and add it to the proposed media mix as an element, and you can run a scenario in 15 minutes. It’s a lot more useful tool to work with real-world changing conditions than the previous, “Hey, here’s your MMM report that you get every six months.
Lauren: Okay. We’ve got a model. What do we need to make the model? Let’s just get that out there. What are the inputs that are needed?
Rich: The basic inputs are, what are you investing in at a channel level, by week, and if you want to, by tactic. Again, your model can get– There’s different levels of specificity in the output. You can just get a model that spits out at the channel level what you should do. You could also get a model that spits out at the channel and the tactic level, but it all depends on what you put in.
Lauren: Channels can include TV, radio, billboards.
Rich: SEO.
Lauren: Other field initiatives, SEO. I want to be clear that although we’re going to call it media mix modeling, the reason we’re calling RevRx™ investment mix modeling is that it’s actually broader than that.
Rich: Correct. You need that. You also need weekly revenue, or you can do an annualized revenue. Weekly revenue is obviously more accurate because you’re getting correlation between expenditure by week and revenue by week. Then you need external factors. Training the model on, this is our peak season. This is when seasonality happens. That’s why the revenue peaks here. This is when we opened a new location. This is when–
Lauren: We brought in a call center.
Rich: Put in a call center, whatever it is, right? The more external factors that you can give to the model so that it can normalize those data points that look like outliers and understand them, the better its predictive nature is, because it’s taking that into account. It’s not just that your media or your channels outperformed in that instance. There was another factor that was driving it. Those are really the main things that you need to put into the model in order to get the right output. Typically, what you’re looking for in terms of a margin of error when you’re thinking about predictability is a good margin of error within 5%. You’re very close to the actuality. You’re at 5% off, give or take either side.
Lauren: In terms of historics, 24 months, right? We really need about 24 months of data to be effective. If you’re in this process now where you’re thinking, “Okay, I’ve had solid tracking for the last 12,” think about MMM maybe being something you’re considering towards the end of 2025. Make sure you’re collecting the data in a way where you have access to pull down that data, kind of on the weekly basis in some of the ways that we spoke about. I want to talk now specifically about RevRx™ and what we mean by investment mixed modeling and not just the inputs into the algorithm.
What are the outputs and how should you be using them? We’ve got all these inputs. We’re then understanding the marginal impact of investing in any given channel, maybe even any given tactic that doesn’t just make your investment inherently work better for you at that point. You have to then know what to do with it. When you’ve got multiple locations, multiple service lines, providers, capacity data, you need to then know how do I use those inputs plus what I know about the effective marginal ROI of my channels to then make the right choices.
Rich: Correct. I would call those constraints. What you’re going to see is if you just put in the inputs that we talked about, the model, if you said optimize the ROI and give me the most optimal ROI projection, it’s probably going to be a lot greater ROI than you’re getting today. That is because there’s no constraints that actually exist in the real world like, “Hey, I only have three providers at this location that I have to support, and even though digitally that location performs brilliantly, they can only see 30 patients a day.” There is a natural constraint. You can’t just drive 500 patients a week to that location because they can’t support it. They can only see 150 of those patients, so the extra 350 don’t get seen.
Those are the constraints that you have to start putting onto the model to help it make better decisions at better levels, taking the business need, but then it should be able to optimize with those constraints in mind. It is essentially optimizing the spend based on those different business units and business needs inside of the overall portfolio of business. If you are multi-location or if you are multi-service line. I think the only thing that you’ve got to think about is once you start segmenting the data, we see this with other issues with optimizing and all sorts of things, once you really start segmenting the data down to a certain level–
Lauren: Like location.
Rich: The data might become less reliable. You can put constraints in the model that will help you to deliver more real-world scenarios that are achievable.
Lauren: Should slicing it up by location not be possible given the effectiveness, still running MMM to understand your channel mix, and then you have a partner or someone internally who is thinking through those constraints, the constraint doesn’t always have to be part of the model. What we’ve found is it can be just as effective to have a human then making those considerations.
Rich: Yes, that’s right. I think also the other thing that human does and that we’re doing on our side is they’re evaluating the current effectiveness of those channels. I think that’s the other key piece with RevRx™ that we’re bringing to the investment mix modeling approach is somebody who is evaluating how mature the channels are in the mix, how far through that optimization curve they are. Essentially what else we can get out of them over time as we continue to test and optimize. You can even put those things in the model, right? You can say, “Yes, paid social, I’m getting a $50 cost per acquisition right now.”
We’ve only just started running it. Based on our experience, we expect to be able to test and optimize to a point where we can lower that by 50%. You can tell the model that actually three months from now, I’m expecting my acquisition costs on paid through the optimization that we make at Cardinal to be half of what it is today, and to factor that in when you’re making projections and investment recommendations based on the mix.
Lauren: So important that point, because we’ve had instances where clients or prospective clients have come to us and said, “I have an MMM solution. This person said that this was the marginal ROI of these channels. We now think we should be investing in X, Y, and Z.” We go and audit those channels and we say, “Wait a minute. This channel is performing at 50% of the efficiency that it could be. Your time over the next six months would be better spent focusing in on repairing this channel, bringing it up to best practice, rerunning the model, understanding then what your marginal investments are and then moving into channel testing.” Just to wrap us up here, Rich, who do you think this type of solution is best for?
Rich: Any client that is spending $60,000 a month on marketing that could go into the investment model.
Lauren: Now, 60 is a good threshold, but if you are doing a hundred million dollars a month, 60 out of a hundred million in revenue is going to be really hard to draw a correlation. So ensuring that the relativity makes sense.
Rich: That is true. That is true. Assuming that you’re following– Like 3 to 5% of your revenue is being reinvested in marketing or more, then that level of expenditure should make you an ideal candidate. Obviously, the more you spend, the better. That’s our goal, right? Is to get you to spend more with RevRx™ because marketing is going to be so much more effective for you.
Lauren: Rich, thanks for joining us. Thank you all for listening to Ignite: Healthcare Marketing Podcast. Share, comment, follow us wherever you’re listening. We hope to see you next time.
Announcer: Thanks for listening to this episode of Ignite. Interested in keeping up with the latest trends in healthcare marketing? Subscribe to our podcast and leave a rating and review. For more healthcare marketing tips, visit our blog at cardinaldigitalmarketing.com.